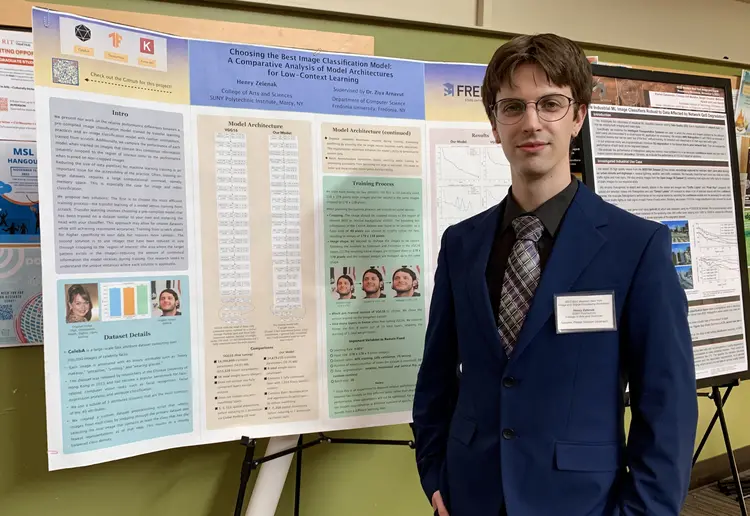
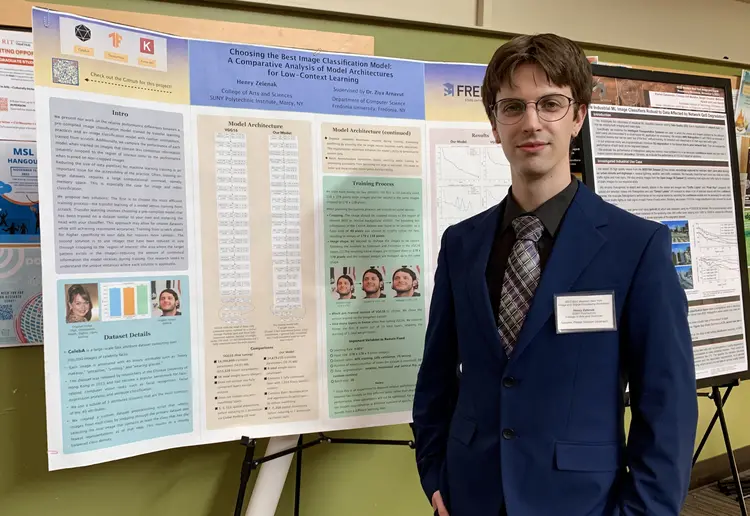
SUNY Fredonia alumnus Henry Zelenak, ’23, with his poster at the IEEE Western New York Signal Processing Workshop.
SUNY Fredonia alumnus Henry Zelenak, ’23, with his poster at the IEEE Western New York Signal Processing Workshop.
SUNY Fredonia alumnus Henry Zelenak delivered a poster presentation, "Choosing the Best Image Classification Model: A Comparative Analysis of Model Architectures for Low-Context Learning,” at the IEEE Western New York Signal Processing Workshop held at the Rochester (NY) Institute of Technology.
Mr. Zelenak, who graduated summa cum laude in May with a B.S. in Sound Recording Technology and a minor in Computer Science, began researching the topic in an independent study during the spring semester, collaborating with Department of Computer Information Systems Professor Ziya Arnavut. The work continued into the summer. The workshop was held Nov. 3.
The study addressed the relative performance differences between a pre-compiled image classification model trained by transfer learning practices and an image classification model with custom architecture, trained from scratch. Zelenak compared the performance of each model when trained on images that contain less contextual information (severely cropped to the region of interest only) to the performance when trained on uncropped images.
“Reducing the size of data pipelines for machine learning training is an important issue for the accessibility of the practice. Often, training on large datasets requires a large computational overhead, namely memory space. This is especially the case for image and video classification,” Zelenak wrote in the study’s abstract.
Zelenak proposed two solutions.
The first was to choose the most efficient training process – the transfer learning of a model versus training from scratch. Transfer learning involves choosing a pre-compiled model that has been trained on a dataset similar to your own and replacing the head with the classifier.
“This approach may allow for smaller datasets while still achieving reasonable accuracies. Training from scratch allows for higher specificity to your data but requires more samples,” Zelenak indicated.
The second solution was to use images that have been reduced in size through cropping to the “region of interest” – the area where the target pattern exists in the image – reducing the amount of contextual information the model receives during training.
“Our research seeks to understand the unique instances where each solution is applicable,” Zelenak wrote.